Patrick Wieschollek
Machine Learning PhD | Senior Applied Scientist at Amazon
Autonomous Robotics / Computer Vision / AWS Cloud Infrastructure
As an Autonomous Navigation & Perception Specialist, previously working on Amazon Scout, with expertise in Machine Learning, Computer Vision, and Massively Parallel Computing, I implement core functionalities for next-generation robots at Amazon. My algorithms are optimized for embedded systems. As a certified AWS Cloud Solution Architect, I further design, develop, and implement complex cloud-based solutions to support internal Amazon projects.
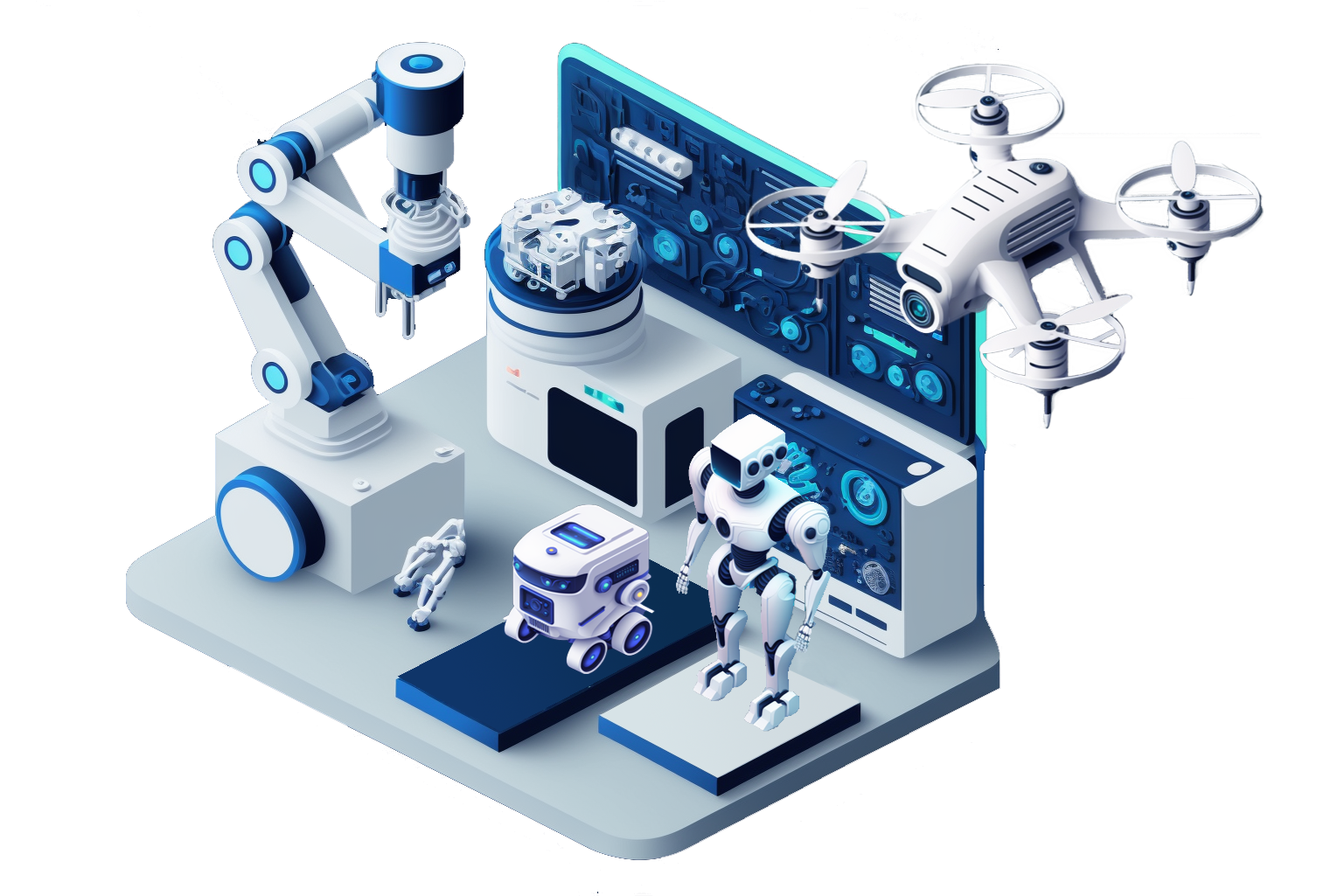